Case Study - Neural Network DC Motor Control
This text explores the possibility of replacing conventional PID controllers in DC motors with neural networks, aiming to achieve autonomous motor control through machine learning. The approach involves training a neural network, with a single hidden layer of seven neurons, on a lightweight microcontroller, resulting in the network effectively serving as a controller for the motor, capable of adapting and learning during normal operation.
- Customer
- ARM Cortex-M0
- Year
- Service
- Research & Development
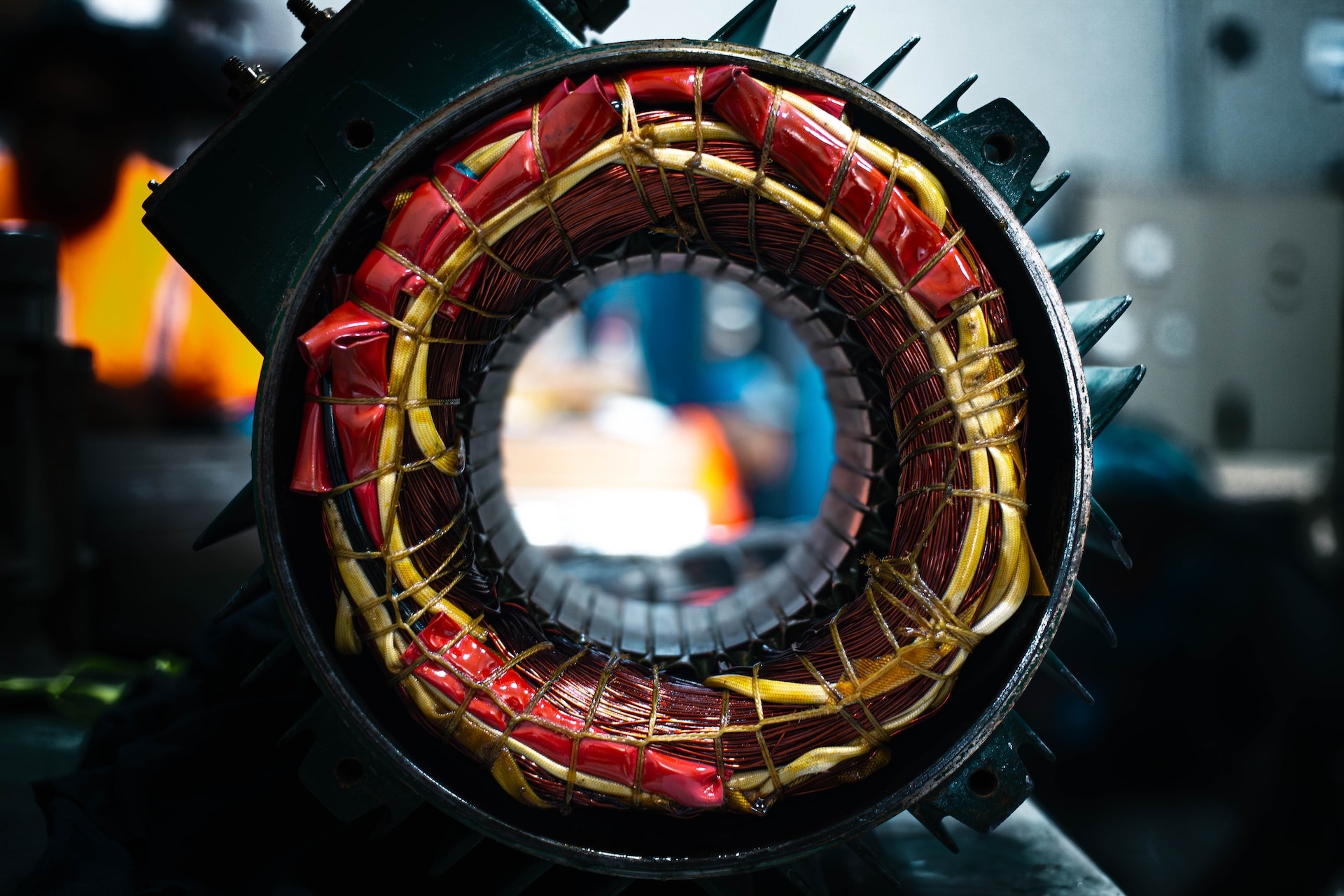
Question: Can we train a neural network on a small ARM Cortex-M0 microcontroller to control the speed of a DC motor?
Answer: Yes, it is possible! A neural network with a single hidden layer can be trained to perform direct inverse control!


With the emergence of new machine learning techniques, the question arises whether it is possible to use a neural network as a replacement for the PID controller. In the best case, the network learns during a training phase how to control the motor by itself. In order to keep the cost low and avoid any additional setup, the training should be carried out on the small microcontroller which is already there. It turns out that this goal can indeed be achieved. The easiest way is to create a neural network which learns to depict the inverse function of the motor to be controlled. After the training, the network can be applied in front of the motor (see image above), which makes the motor follow any given speed reference.

In experiments, this has been achieved using a neural network with a single hidden layer consisting of seven neurons. During a short training phase (~12 min), different voltages and loads are applied to the motor. Afterwards, the network can be applied in inference as a controller. It can even be extended in a way, that the network continues learning during normal operation.
For more information, see
Funk, F., Bucksch, T., & Mueller-Gritschneder, D. (2020). ML Training on a Tiny Microcontroller for a Self-adaptive Neural Network-Based DC Motor Speed Controller. In IoT Streams for Data-Driven Predictive Maintenance and IoT, Edge, and Mobile for Embedded Machine Learning (pp. 268-279). Springer, Cham.
What we did
- Analysis
- Research
- Documentation
Replacing traditional PID controllers in DC motors with neural networks is a revolutionary approach. By training a neural network on a lightweight microcontroller, like ARM Cortex-M0, we can achieve cost-effective and adaptive motor control without manual tuning. The key is a short training phase where the network learns the inverse function of the motor, ensuring efficient performance even during normal operation.

Author
- Inference Accuracy
- 95%
- Resource Utilization
- 1 MB
- Training Time Efficiency
- 12 minutes